O have you caught the tiger?
And can you hold him tight?
And what immortal hand or eye
Could frame his fearful symmetry?
And does he try to bite?
Yes, I have caught the tiger,
And he was hard to catch.
O tiger, tiger, do not try
To put your tail into my eye,
And do not bite and scratch.
Yes, I have caught the tiger.
O tiger, do not bray!
And what immortal hand or eye
Could frame his fearful symmetry
I should not like to say.
And may I see the tiger?
I should indeed delight
To see so large an animal
Without a voyage to Bengal
And mind you hold him tight.
Yes, you may see the tiger;
It will amuse you much.
The tiger is, as you will find,
A creature of the feline kind.
And mind you do not touch.
And do you feed the tiger,
And do you keep him clean?
He has a less contented look
Than in the Natural History book,
And seems a trifle lean.
Oh yes, I feed the tiger,
And soon he will be plump;
I give him groundsel fresh and sweet,
And much canary-seed to eat,
And wash him at the pump.
It seems to me the tiger
Has not been lately fed,
Not for a day or two at least;
And that is why the noble beast
Has bitten off your head.
Platypus Header
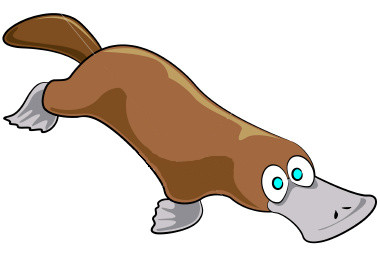
Platypus Innovation Blog
29 December 2016
16 August 2016
Computer Generated Haiku - a project by Aji Alham Fikri & Daniel Winterstein
Here are a couple of write-ups of the computational creativity in poetry research that Aji and I did last year:
I'm planning to extend this into a general purpose poetry generator / evaluation. You can see the work-in-progress notes for that here: a JSON specification format for poetry
I'm planning to extend this into a general purpose poetry generator / evaluation. You can see the work-in-progress notes for that here: a JSON specification format for poetry
22 June 2016
Agile Procurement?

Image from Brazil, a gloriously dark comedy about bureaucracy and power by Terry Gilliam. Actual relevance to this post: low, but I like the movie.
Partly, because high-profile software projects are difficult, and public-service software often has to handle lots of corner-cases that make off-the-shelf solutions harder to use. But partly it is their own fault: Public procurement sets up systems that almost ensure they will pay too much for second-rate software. Why?
One such model is the framework agreement. Companies first tender to be on the list to tender for actual work. Bureaucratic framework agreements create an overhead that eliminates most small software companies (who are of mixed quality but contain many of the best developers) in favour of large contractors (who tend to charge more, often a lot more, and deliver older and less flexible software).
I expect the bureaucracy is trying to manage risk & overhead: Do some heavy vetting once, then re-use it. But this vetting is of little value. A large contractor will submit their successful past projects, possibly carried out by teams who have no connection to the teams that will then work on the tender. A small contractor has less track record to draw on, so is at a disadvantage. Inspite of the care taken by procurement, government software projects often over-run or fail to deliver. Inspite of... or because of?
There are better ways to manage risk in software projects! We need Agile Procurement. Not procurement of agile software, but agile ideas in procurement itself. That is, procurement teams who work iteratively with the supplier and consumer teams, taking small short-term risks as the best way to manage costs and avoid large risks.
I'd also like to see multiple redundancy in procurement. Instead of betting everything on one big contract with one supplier... have several suppliers produce prototypes, at least for the early stages. If one supplier fails to deliver -- it's not a problem. This would allow for lighter touch procurement -- opening the door to SME software development companies. Given the difference in costs, I believe this would actually lower the overall price. It also allows more ideas to be explored, and it introduces some post-tender competition -- and hence better software at the end.
10 May 2016
A Simple Intro to Gaussian Processes (a great data modelling tool)

A Gaussian Process, fitted using MatLab, showing most-likely-value & confidence interval. Note how the shape hugs the data, and how the uncertainty varies depending on the data - sometimes the model is confident, sometimes it isn't, and you know which is which.
Gaussian Processes (GPs) are a powerful technique for modelling and predicting numerical data. Being both relatively new and mathematically quite complex, they're not as well known as other techniques. They have some strong advantages:
- Flexible: Can be used to model many different patterns.
- You make fairly few assumptions about the model.
- Based on probability theory, so they have a solid mathematical grounding.
K Nearest Neighbours (KNN)
K Nearest Neighbours is a classic AI algorithm, and very easy to understand. It also provides a surprisingly good basis for explaining Gaussian Processes (without any maths involved yet). Here's how KNN works:The task: Given an item x, predict the category of x. E.g. you might ask, Is this email spam or not-spam?
The method:
- Let's pick k=5.
- Given an input item x... E.g. a fresh email has arrived, is it spam?
- Look through your training data, and find the 5 items most similar to the input item x. These are the nearest neighbours.
- Look at the categories those 5 items have, and predict the most common category from them.
- Done :)
- It is a lazy algorithm. The model isn't trained in advance, as with say Linear Regression or Naive Bayes -- instead the work is done when an input item is presented.
- The data is the model. If you have enough data, KNN can model a really wide range of patterns. Rather than being forced into a particular shape, the data can speak for itself. There is a cost to this though - it requires more training data.
- The key part is judging when items are similar. How you do that will depend on the problem you're looking at.
The classic KNN algorithm is for predicting categories (e.g. spam / not-spam), but we can modify it as follows to make numerical predictions (e.g. the price of fish):
Step 4': Having found the k nearest neighbours, take the average value.
From KNN to a Gaussian Process
So what is a Gaussian Process?It deals in numerical data, e.g. the price of fish -- and for the rest of this article, let's say it is the price of fish as a function of date that we're modelling. We keep all the training data, and say that when predicting new data points from old ones, the uncertainty/noise will follow a multivariate Gaussian distribution. The relationship given by that distribution lets us make predictions from the training examples.
For non-Mathematicians, let me briefly cover the terminology from that paragraph. A distribution tells you how likely different values are. The Gaussian Distribution, aka the Normal Distribution, has a bell-shaped curve: the mean is the most likely point, and the probability drops off rapidly as you move away from the mean. The multivariate Gaussian (which is what we want) can specify correlations between multiple variables. The Gaussian Distribution naturally arises in lots of places, and is the default noise model in a lot of machine learning.
Similar to KNN, the Gaussian Process is a lazy algorithm: we keep the training data, and fit a model for a specific input. Also like KNN, the shape of the model will come from the data. And as with KNN, the key part is the relationship between examples, which we haven't defined yet...
Introducing the Kernel Function
A Gaussian Process assumes that the covariance between any set of points is a multivariate Gaussian.The "variables" here are the different values for the input x. In our price-of-fish example, the input is the date, and so every date gives a dimension! Yes, in principle, there are an infinite number of variables! However we only have a limited set of training data -- which gives us a finite set of "variables" in the multivariate Gaussian -- one for each training example, plus one for the input we're trying to predict.
A multivariate Gaussian is defined by it's mean and covariance matrix, so these are the key things for us to calculate.
The kernel function of a GP says how similar two items are, and it is the core of a specific Gaussian Process. There are lots of possible kernel functions -- the data analyst (e.g. you) picks an appropriate one.
The kernel function takes in any two points, and outputs the covariance between them. That determines how strongly linked (correlated) these two points are.
A common choice is to have the covariance decrease as the distance between the points grows, so that the prediction is largely based on the near neighbours. E.g. the price of fish today is more closely linked with the price yesterday than the price last month. Alternatively, the kernel function could include a periodic part, such as a sine wave, to model e.g. seasonal ups and downs. The Wikipedia article lists some example kernel-function formulas.[1]
The kernel function will often have some parameters -- for example, a length parameter that determines how quickly the covariance decreases with distance. These parameters are found using optimisation software -- we want parameters that optimise the likelihood of the observed data. We can write down the probability of the observed data (the likelihood) as a function of the kernel function parameters, and then pick kernel function parameter values to maximise the likelihood.
From the covariance matrix, you can then calculate the prediction for x. The prediction for x (or in probability theory terminology, the marginal distribution for x) will be a simple one-dimensional Gaussian. It has a mean value (which is the most likely value for x) and a standard-deviation for the uncertainty.
Building a GP
This article has skipped over the technical details of how you carry out certain steps. I've blithely written about "optimising the likelihood" without saying how you do that. That's partly because there are multiple ways, and partly to keep this article simple. The short answer is: you'll be using software of course, and most likely software that someone has kindly already written for you.I'm not going to recommend a particular software tool here, as the choice really depends on what you're familiar with and where you're using it. There are GP calculators available for many environments, e.g. Weka has one for Java [2], or you can talk to your local Winterwell office[3] :)
Going Deeper
[1] Commonly used kernel functions, in Wikipedia: https://en.wikipedia.org/wiki/Gaussian_process#Usual_covariance_functions[2] Weka, a Java toolkit for machine learning.
[3] Winterwell, data science consultancy
So you want to know the mathematical details? Good for you!
Try reading these resources:
[4] "Gaussian Processes: A Quick Introduction" by Mark Ebden.
[5] "Gaussian Processes for Machine Learning" by Carl Rasmussen and (my boss once-upon-a-time) Chris Williams.
1 April 2016
“Have a Nice Day!” smiled the robot
Automation makes it easy to spam people. Makes it easy for normal business-people — people who are quite genuine in their day-to-day dealings — to spam people.
Part of the problem is the curse of subjectivity — their email is spam, my message is an valuable communication. Caught up in our own projects, it’s easy to forget that people receiving the email/sms/tweet may not care.
But that kind of unwanted message is relatively forgiveable. There is a worse kind — the message that pretends to be something other, to worm it’s way past your defences. The insincere message. And it’s surprisingly easy to write one,
The main culprit is the advert that doesn’t acknowledge that it’s a sales message, but pretends to be something else — helpful or part of a conversation. My inbox is full of these.
- “Are you validating performance for your mobile users?”
- “Re: Making Great Customer Experiences”
Double spam points there! Pretending to be a reply to me, plus a subject line that doesn’t acknowledge it’s an ad. - “Daniel, open this email for 12 people you should meet :)”
Sure, the cheap trick of using my name did help them get noticed – but to what end? - “I want you back for good”
Suggestive for a B2B message! But pretending to be on friendly terms with a stranger is insincere. It doesn’t make the message cute, it makes it annoying.
Straight to spam! And when you do read one of these? Disappointment lies ahead: the headline makes click-bait promises to lure you in, but then post does not deliver. You’re likely annoyed at having been fooled into a click — hardly the best start to a customer relationship. If a company starts the conversation with an insincere email — do you trust them?
As a contrast, here’s a sincere sales message:
Network @ BDX Glasgow Next Week (And Win Your Own Office!)
Good honest work BDX: It’s clear from the subject that this is an ad for a Glasgow networking event. If I’m interested, I’ll read it. It is an advert, but that's OK.
In real life, if someone behaves insincerely, soon enough they notice people avoiding them. With digital we may not notice the bad impression insincerity leaves. And with automation… Automation can amplify the problem. With automation, one can be insincere at scale. Pushy insincere tactics can get more clicks. But click-counting doesn’t measure that it also annoys. The pushy marketer doesn’t see the people who don’t click. This can lead to companies blindly optimising for spam, and trashing their own brand.
The problem is not automation, nor is it measurement and optimisation — these are tools that can amplify an underlying problem. The problem is a lack of sincerity.
Here’s a simple test of sincerity: If the recipient knew the full story behind the message — how the recipient was chosen, how the message was crafted, and what the sender hopes to achieve — Would that affect how they read it? If so — some insincerity may have crept in.
So: At SoGrow we’ll be trying to keep it sincere. When one of our bots talks to you, they will hopefully act in a sincere manner — e.g. being open when doing sales.
Yours sincerely,
– Daniel
– Daniel
Should charities share profile data, after the tragic death of Olive Cooke?
The Olive Cooke case is a striking example where an individual who cared enough to take an interest in charities can end up being bombarded with mail. The case became more dramatic with claims that Olive Cooke was actually killed by this, although the family vociferously denies this.
There has been much soul searching since, and Third Sector magazine has pointed out that 99 charities had Olive Cooke's contact details, of which 16 failed to provide any opportunity to opt out, 56 required her to proactively contact them if she wished to opt out, and only 14 provided an opt-out tick-box.
It is natural to want to curb the data sharing that led to Olive Cooke receiving so much mail, and I don't want to discourage the valuable measures that help people better understand what happens with their data.
However are calls for charities to avoid using profile data the right reaction?
The average person is bombarded by marketing, with charities only being a fraction of the problem. Other mailings are often more dangerous and certainly less defensible. It would be perverse to rule that charities must limit their marketing, whilst giving free rein to all manner of profit-focused companies. That includes more widely dangerous material, such as seductive loan offers, from the credit card offers banks bombard us with through to the more obviously unscrupulous pay-day lenders.
In principle, there are already tools to protect you from unwanted contact. The Mail Preference Service, Telephone Preference Service, and Email Preference Service provide a way to opt out of cold-calls and junk mail. But for the charity marketing industry to point to these as solutions is not good enough. Most people do not know of these. Also many organisations do not check against these lists (it is not straightforward, and there is no free service to do so). Finally, these opt-outs do not restrict contact from organisations who can claim a connection (e.g. from a previous contact, which might be as little as an online petition), or where you have, often accidentally, consented to your data being shared.
We might ask charities to employ more care. But how can a charity really screen large mailing lists, and cross-check with other charities? The admin challenge there is large and complex. If charities were to spend on this, they would find themselves even more criticised for high admin costs.
We might urge self-restraint -- but it is unrealistic to expect self-restraint from marketing teams to be sufficient. Even where marketers have the best of intentions, their position is too subjective -- “other organisations send junk mail; our messages have valuable information of hopefully mutual interest.” Nor would we want charities to act in a half-hearted one-arm-behind-their-back manner -- we want charities to be as effective as possible, and that includes efficient marketing and effective fund-raising.
What is the way forward for the Third Sector?
It begins by acknowledging there is room to improve practices in charity marketing, and following the death of Olive Cooke, a real need to improve. That does not mean avoiding modern marketing tools. Turning back the clock is rarely the answer. Instead of asking charities to be less-effective, we should establish best-practice guidelines, educate the sector about them (which includes educating more charities on how to effectively use these tools), and ensuring best practice is adopted and adhered to.
Let us suggest some concrete measures charities should adopt in their use of profile data:
- It must be easy to opt-out of communications.
- If consent is withdrawn, that should be passed on to any partners with whom the data has been shared.
- Rather than having to opt-out from every individual charity, it should be possible to opt-out of communications from whole sectors.
- Consent to sharing profile data must be genuine -- the current practice of sneaking in a tick-box is not acceptable.
Longer term, could data be managed better to give a more holistic view of communications with the person, with trusted bodies mediating marketing? Such bodies could better understand whether someone was being bombarded with messages from other charities, and understand whether someone has a specific cause they are interested in, in which case they wouldn't want to hear from other charities in other areas. Or indeed whether someone is just not interested in giving to charity at all. This would be good for the charity, because it can reduce their mailing costs and allow for more personalised messages, and good for people like Olive Cooke too.
Written with Sanjay Joshi for sogive.org
3 February 2016
Neal Stephenson on corporate structures?

Out-sourcing, automation, and online sub-contracting are all chipping away at the traditional company. Today small start-ups can explode into worldwide disruption. Looking forward -- will this only increase? With ever-smaller core teams acting as the focal point for powerful flows of money and services. It makes me think of this passage from Neal Stephenson's The Diamond Age where the book's second hero, John Hackworth, travels through the Coastal Republic, a decadent country propped up by high-technology:
The Coastal Republic checkpoints at the intersections of the roads were gray and fuzzy, like house-size clots of bread mold, so dense was the fractal defense grid, and staring through the cloud of macro- and microscopic aerostats, Hackworth could barely make out the hoplites in the center, heat waves rising from the radiators on their backs and stirring the airborne soup. They let him pass through without incident. Hackworth expected to see more checkpoints as he continues toward Fist territory, but the first one was the last; the Coastal Republic did not have the strength for defense in depth and could muster only a one-dimensional picket line.
Subscribe to:
Posts (Atom)